Mon Jan 27, 2025
AdaptoFlow: Adaptive Sensing & ML Inference on 5G/6G deployments
AdaptoFlow is a cascade project of TrialsNet EU project and aims at upgrading TrialsNet’s use cases, namely UC1 – smart crowd monitoring and UC4 – smart traffic management in smart cities, through advanced AI/ML techniques, 5G networking, and edge computing infrastructure. AdaptoFlow aims to transform the current monitoring and AI/ML inference paradigm from a traditional, centralized approach to a dynamic, decentralized one that leverages in situ data analysis at the edge of the network.
The focal point of AdaptoFlow is to introduce a service supporting and enriching AI/ML inference and data stream processing for applications deployed in geo-distributed realms so that data movement, energy consumption, and app-level latency are reduced by adapting the intensity of the execution at runtime taking into account the evolution of IoT generated data and the state of the underlying computational resources of edge nodes, while maintaining acceptable QoS guarantees requested by application operators. In a nutshell, AdaptoFlow will support the UCs with data-driven intelligence in the form of:
-
adaptive data stream processing, where AdaptoFlow will output runtime suggestions to the monitoring module of the UCs for what the monitoring intensity (data collection periodicity and dissemination) should be. This will be performed by monitoring the data stream behavior dynamics of the numerical data extracted by the wifi access points, so that the intensity at which the data consumed to output crowd analytics (i.e., people counting) is dynamically adjusted. This will reduce the volume of data that must be processed and provide “breathing space” to the analytics modules during phases of low volatility in the data behavior dynamics;
-
energy-aware model swapping, where AdaptoFlow will aid Edge AI services in providing a suggestion so that in the presence of limited resource capacity, the AI/ML model in use can be swapped on-the-fly with another readily available, but less complex, model. With model swapping, AI services can achieve energy savings by employing less computational effort for runtime inference tasks and at the same time, user experience is not negatively impacted with latency bounded by using a less complex model. The key for the AdaptoFlow intelligent mechanisms will be to reduce resource wastage and improve service responsiveness, while at the same time, bound response uncertainty to acceptable user-desired levels.
The key for the AdaptoFlow intelligent mechanisms will be to reduce resource wastage and improve service responsiveness, while at the same time, bound response uncertainty to acceptable user-desired levels.
Keywords: Adaptive Monitoring, Adaptive Sensing, MLOps, EdgeAI, Runtime Alteration, Energy Consumption
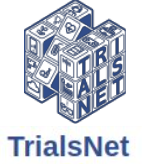